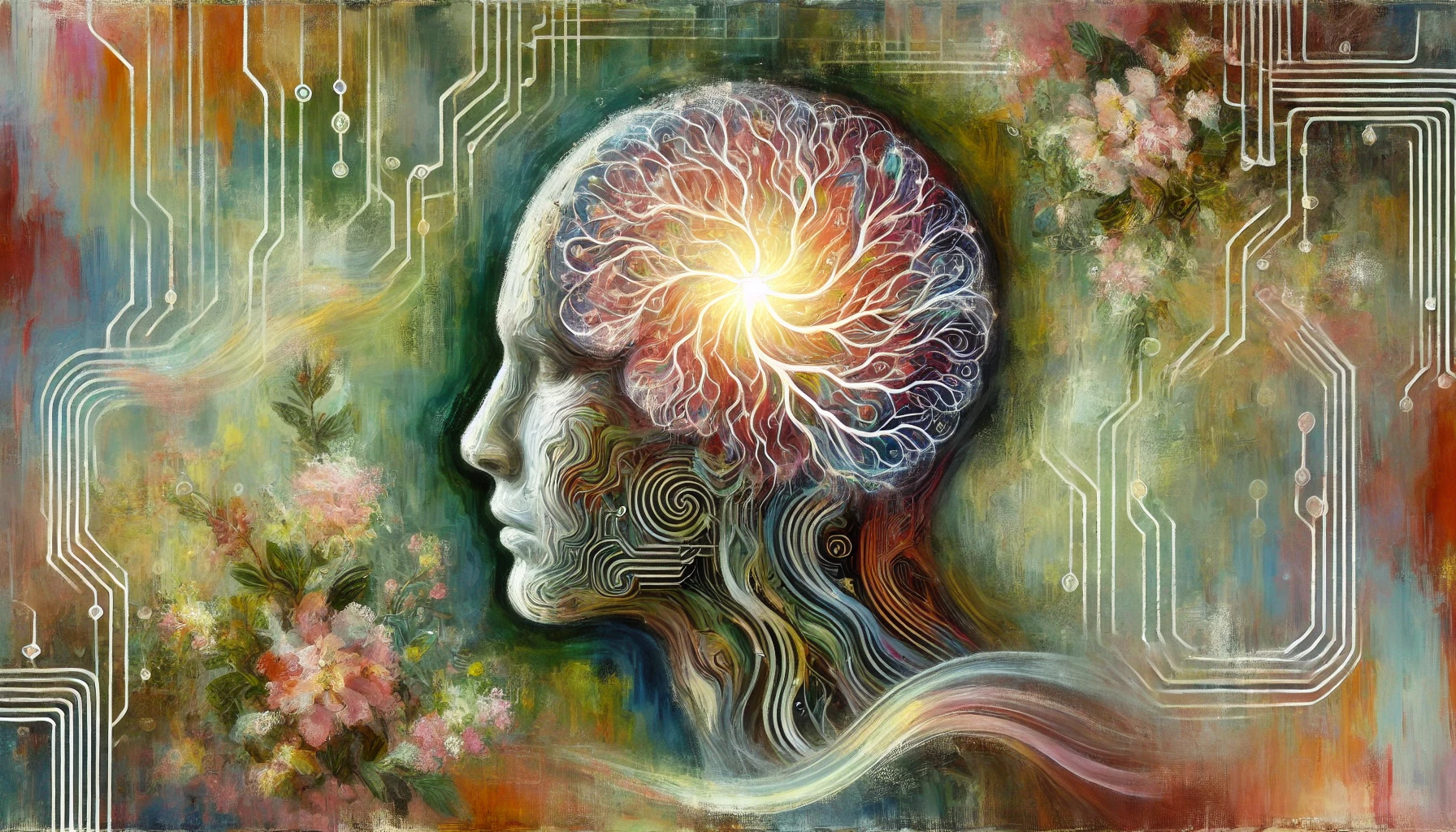
AI discussions span everything from utopian promises to technical deep dives. As someone whose career has centered on observing how humans interact with technology, I’ve witnessed how our systems shape—and are shaped by—our lives. Recently, as a strategist and researcher, I’ve been more deeply exploring generative AI’s potential.
One realization stands out: We urgently need human-centered design (HCD) and established user-centered methods to steer AI’s broader societal impact. By adapting UX principles and embedding them into the foundations of these models, we can limit further entrenching existing divides, biases, and superficial interactions. This article isn’t a technical exploration of generative AI; it’s an open invitation to rethink how we might design it with greater intention.
It’s tempting to believe that AI could be humanity’s next great leap forward—a technology that will profoundly and ethically enhance our lives. In a perfect world, AI would evolve through global cooperation, with governments and organizations collaborating to set agreed-upon boundaries in our long-term interdependence with this technology.
Let’s face it, that’s not going to happen.
AI is already deeply enmeshed in profit-driven models, and the commercial forces pushing AI’s rapid development are unlikely to yield a blue-sky vision of AI as a mission-driven, human-first global endeavor. We live in a world where technology will be monetized as quickly as possible, even if it’s still half-baked, and the long-term consequences still need to be considered.
So, what do we do? Our challenge becomes how to guide generative AI’s development without totally abandoning human values while acknowledging its commercialization.
Given this reality, we start by changing the discourse around AI to embrace its potential and risks instead of sensationalizing them. As we develop these systems, we must ensure their training captures and nurtures the best aspects of who we are. To do that, we must create a common framework for what these “best aspects” are while acknowledging that this will include a wide range of relative truths.
Exploratory research asks big questions. Are we taking necessary steps to ensure AI is optimized to enhance humanity’s richness and complexity, or are we reducing ourselves to commodified data points that reflect only the most sensationalized aspects of our behavior? This question should be at the forefront to avoid repeating mistakes.
Digital’s Complex Legacy
From my early work in broadband development to today, I’ve watched well-intended, beneficial advancements also lead to long-term, harmful consequences. Much like current promises about AI, broadband was initially seen as a democratizing force—a tool to empower and connect. High-minded ideals and a utopian vision drove the people who built these services. I know as I was one of those people. I’ve struggled with the pride of being part of an exciting time in our technological history and disillusionment with how some aspects have turned out. As commercial interests took over, particularly with adopting the ad-driven model, the Internet’s original promise was transformed.
Many of the unintended consequences we are dealing with now were foreseen by many, but it didn’t matter; the dot-com bubble burst created an existential crisis for the industry. It forced profit-or-perish decisions that shaped much of today’s Internet, turning hopeful optimism into a commercial necessity. The same unchecked optimism and as much doomsday prediction now surround AI. Might we use AI development as an opportunity to address the negative aspects of our past technological choices head-on?
Due to how broadband shifted to survive, generative AI has been given a complex inheritance. Profit-optimized content has been the foundation for its simulated understanding of human interaction.
Shallow Data In, Shallow Interactions Out
Too often, technology has forced us to adapt to its limitations rather than expand ours. An attempt to address this was in early advocacy for natural language interfaces—aligning systems with how people organically communicate and making interactions easier rather than forcing users to conform to rigid, efficiency-driven workflows. AI should be no different, especially given its potential to be deeply woven into our daily lives.
Today, most AI systems are built on data from platforms like Google, Facebook, and TikTok. These platforms prioritize engagement, rewarding the most attention-grabbing content, not necessarily the most meaningful. While vast and seemingly comprehensive, it represents inaccurate and incomplete versions of ourselves. Consider for a moment those sources. Do they reflect our goals as a species?
Some AI proponents believe techniques like cross-domain learning and transfer learning—where AI systems are trained on data from multiple domains—can mitigate biases and data gaps. These techniques can improve AIs’ ability to handle more complex tasks. However, these technology-focused solutions are band-aids unlikely to address the underlying design failure: the data itself.
One of my favorite tech dad jokes came from a colleague at a systems integrator: “How did God create the universe in 7 days? There was no legacy system.”
I’m passionate about technology, but we must be pragmatic in its implementation, and the devil is in the details. Surrounded by the hype of AI, it’s easy for tech leaders to forget: Legacy systems have taught us once systems are implemented, they tend to persist, flaws and all. Retooling from scratch is costly and time-consuming, meaning the foundations of today’s AI systems will continue to shape tomorrow’s world. Without a focused effort to integrate context-rich, qualitative information, we risk building AI systems that fail to enrich our lives, limit how we engage with the world, and codify narrow representations of ourselves well into our children’s futures.
We’ve Already Adapted to Technology’s Distorted Lens
Seemingly insignificant behaviors can reveal profound insights that broad datasets miss. Hesitations, pauses, or other external behavioral changes could indicate a point in a workflow that inherently requires a higher cognitive load or a genuine barrier to completing a task—big data, which typically only captures outcomes, fails to detect this. There is an even more concerning fact: users often adapt to poorly designed system experiences and believe their struggles stem from their inadequacies rather than the technology itself.
We’ve watched technology move beyond being a tool we adapt to in small ways into a force that shapes our culture in significant ways we don’t control, eroding our collective feeling of agency. Narratives pushed by the algorithms behind these platforms don’t just diminish our senses of self-worth and reality but also encourage consumerism, impulsivity, and emotional reactivity. As AI systems inherit data from these platforms, they reinforce these interactions, codifying hyper-consumerism, performative relationships, and attention manipulation.
Our lived experiences—unique, messy, and deeply meaningful—are being flattened, standardized, and spit back at us in increasingly distorted forms. Not only are we facing a loss of biodiversity but also of human diversity, and it’s unfolding on our watch.
Correcting Course
At the heart of AI’s current development lies a mismatch between its commercial incentives and society’s broader needs. The race to deploy AI to boost stockholder confidence undermines its real potential. Yet, we can correct course, making conscious choices to align AI’s evolution with our better angels.
Pivoting to a Long-Term Investment Model
We should adopt a long-term investment model that encourages careful consideration before releasing AI technologies to the public instead of using us as experiments and training fodder. It’s tempting to think that if we need more data, we can simply allow users to provide it in real-time. However, this data is a feedback loop of simplified tasks and interactions, and I question the ethics of this approach.
Companies can still find a win-win. Jeff Bezos’s well-known approach to Amazon is a powerful example of this kind of long-term investment thinking. From the outset, Bezos emphasized long-term infrastructure over immediate profits, understanding that the company could grow exponentially by building the necessary foundation.
Ethical Walled Gardens
Another crucial component of redirecting AI’s future is the concept of ethical walled gardens. “Walled gardens” often mean monopolistic ecosystems controlled by tech giants like Google or Facebook, but an ethical walled garden can have different purposes. Secure, gated spaces where clear moral principles and safeguards govern AI development and deployment.
Privacy-focused laws, such as the European Union’s General Data Protection Regulation (GDPR), help protect user privacy and create secure spaces for data collection. However, they will not inherently address the quality or completeness of AI training data. While these frameworks safeguard data from exploitation, they must be paired with strategic efforts to improve the collected data.
The Value of Qualitative Data
Addressing AI’s inherited blind spots to build better systems indicates investing heavily in purpose-generated qualitative data such as ethnographic studies, contextual observations, and curated sociological findings. Valuable insights can come from methods that capture real-life, context-rich interactions beyond the direct influence of consumerism. Armed with this goal-focused content, AI can begin to internalize the diverse and frequently counterintuitive ways humans engage with the world, providing essential balance.
To ensure AI systems are trained on meaningful data, we should continue to optimize them to incorporate and use unstructured data more effectively. This will require AI models that can contextualize human behavior. Some research suggests that combining big data with smaller, qualitative datasets—often called data triangulation—can help AI systems better reflect the richness of our experiences.
Generative Qualitative Research for AI
Qualitative data is used today to augment and refine AI models; this is not new. However, this unstructured data (an estimated 80% of all content available) isn’t purpose-built for the role. As discussed, it is subject to the same biases and blind spots as other data. Qualitative data requires significant human effort to clean, code, and curate. A partial solution currently being explored uses a hybrid model, where researchers use AI tools to help codify the data. The importance of human oversight cannot be overstated in this effort.
A complementary and amplifying approach to mining our unstructured data treasure trove is to conduct targeted generative studies that can be more heavily weighted to assist AI models in deciphering the raw data they consume. Rigorous methodologies and research objectives based on collectively defined goals can ensure that specific concepts and dynamics the AI model is trained on are conveyed in a way that requires less human oversight in the long term.
A framework for generative research for AI model training can and should be defined. Methods used in traditional software systems today can provide insight. Foundational principles for current user research are one-on-one interviews (vs. focus groups) and a heavy focus on observational and contextual methods. When properly structured, implemented, and analyzed by people with expertise in human-centered design, this type of research can be highly directional and capture a wide range of targeted, nuanced insights. The results of these studies, codified with the help of AI and weighted by humans, could provide robust training benchmarks.
As we shape the future of AI, let’s move beyond technological optimism or doomism, roll up our sleeves, and work to address our industry’s complex legacy. The time for developing mutually defined goals for human-centered design in AI is now. We have a choice: allow this technology to evolve only to reflect what’s commercially viable or intervene to ensure AI represents us authentically. Answers lie in investing in richer data, focusing on human experience, and refusing to let AI reinforce a flaw that has plagued our digital spaces for decades.
Would you like to learn more about human-centered design and AI? Check out the following materials:
- What is Human-Centered AI (HCAI)? – Interaction Design Foundation: Learn more about HCAI and its ethical foundations in AI development.
- A Human-Centered Approach to the AI Revolution – Stanford HAI: Stanford’s perspective on the importance of human well-being in AI’s future.
- Qualitative Analysis for Human-Centered AI – arXiv: A dive into how qualitative data can improve AI systems and make them more human-centered.
- Enabling Human-Centered AI: A Methodological Perspective – arXiv: A comprehensive framework for integrating human-centered perspectives in AI systems.
About the Author
Dorothy is a digital strategist and researcher who works with companies to blend human-centered research with emerging technologies to navigate complex challenges.